5 Best Practices for Bank Fraud Detection and Prevention
5 Best Practices for Bank Fraud Detection and Prevention
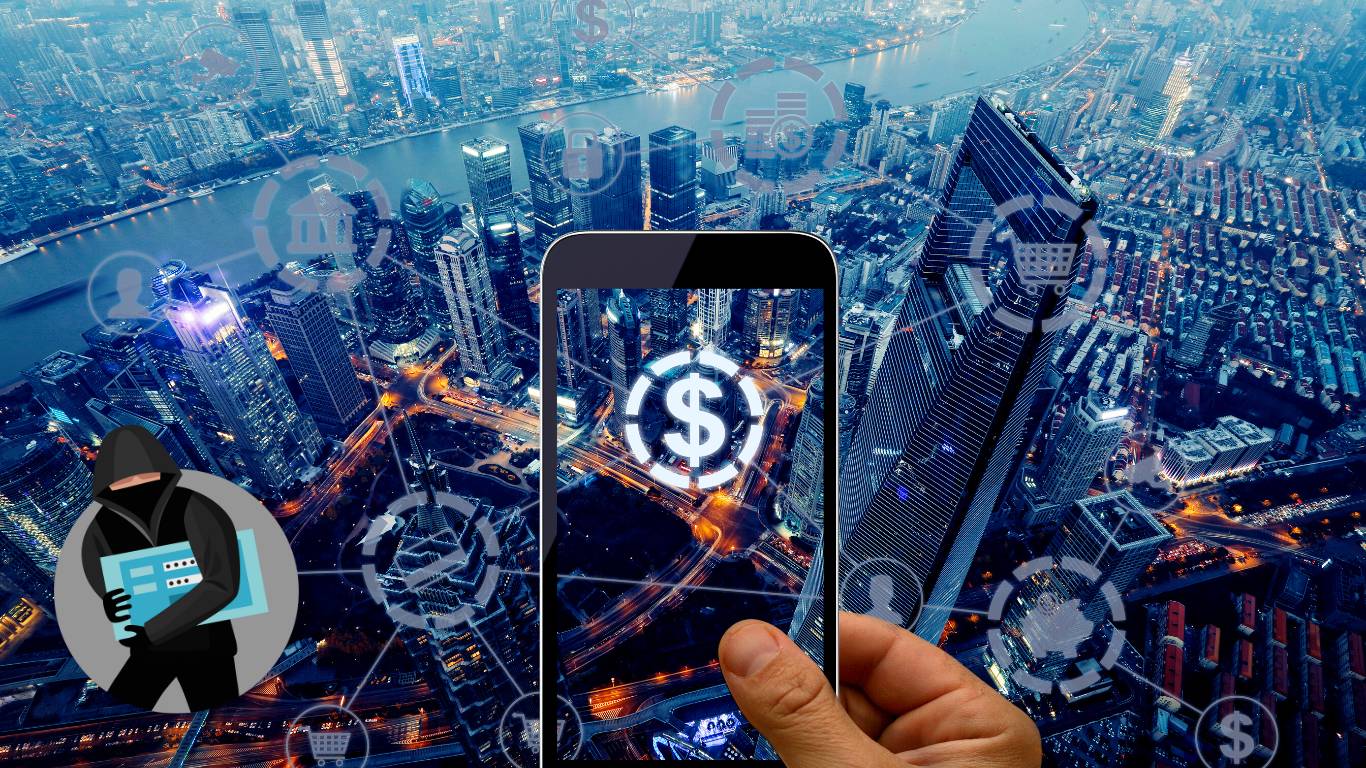
Mar 09, 2023 5:16:52 PM / by Macy Choong
“Government is strengthening measures for bank fraud prevention/ security for digital banking…”, I bet you have seen this somewhere in news or press release during the recent years for sure. As the threats of fraud continue to evolve all around the world, it’s becoming increasingly clear that traditional methods of fraud detection are no longer enough. Not only that, the cost of fraud to financial institutions and the customers is staggering, and the consequences of a successful fraud can be devastating. Banks and financial institutions must take action to stay ahead of the curve. The time to act is now before becoming the next target of the fraudster while the use of the right advanced technologies could make you win the fraud “war”. Here are the best practices that financial institutions should be acting now to enhance their bank fraud detection and prevention efforts while keeping the customers in a highly- secure transaction environment from the never-ending fraudsters attack.
Most Common Traditional Bank Fraud Detection & Prevention Approaches
- Rule-based systems – Use predefined rules to detect fraud, for example, showing alert when a transaction is exceeding above a certain unusual amount.
- Human review (e.g., internal audit or external audit) – Manual examination of transactions and organisation data to find out potential suspicious transactions and take prevention actions.
- Statistic models – Use historical data to identify patterns and anomalies that may indicate fraud.
Problems in traditional Bank fraud Detection/ Prevention
- Not able to keep up to new type of frauds on time – Only able to detect know fraud types. The fraudsters are always looking for new ways to tackle the traditional models, once they found a gap from the models or systems, they will happily take as much money as possible. The banks normally will only find out when unusual huge loss being incurred while this resulting in bad press that might negatively impact customers’ trust on their services and products.
- High percentage of false positivepositive (where legitimate transactions are incorrectly flagged as fraudulent) – lack of relevant data to generate accurate insights to detect frauds and/ or human errors that waste time and effort on exanimating the false positives cases. Research found over 95% of system-generated alerts are closed as “false positive” since 5 years ago and this issue still remain unsolved for many banks nowadays globally.
- Causing bad customer experience – difficulty in balancing fraud detection and prevention with customer experience, as some fraud detection measures can lead to increased friction and inconvenience for customers
- Concerning on compliant issues with regulations – afraid of taking new digitalisation move and/ or having difficulty in searching trustworthy advanced technology providers that able to stay complaint with regulations such as data privacy laws, while collecting and using data for fraud detection purposes and deal with data security and privacy concerns as sensitive customer data must be protected while being used for fraud detection.
Five Bank Fraud Detection & Prevention Strategies that Banks and Financial Institutions Should be Taken
- Artificial Intelligence (AI) and Machine Learning (ML) Algorithms that can identify patterns and anomalies in real-time transactions. The keys of achieving highly accurate fraud detection are having a comprehensive platform or system with
- the ability of intelligent use of data
- the ability to acquire while mash up structure and unstructured data, and
- big data analytics that could analyse large amounts of data from multiple source
These technologies not only can be trained on historical data but also able to learn from the latest data that are being acquired and consolidated though supervised and unsupervised learning algorithms in nearly real time to identify and detect known and new forms of frauds. It also allows the financial institutions to gain predictive and actionable insights about consumer digital footprints and the latest fraud trends.
- The Use of Behavioural Data & Behavioural Targeting Technology can reduce fraud by analysing and identifying unique online behaviour patterns of customers. In this fast-changing environment, it’s important to understand the customers by comparing the customer's current behaviour to their typical behaviour through behavioural science>. A better understanding about the customers behaviours could increase opportunities to optimise customer experience while also to discover suspicious patterns for fraudulent activities. Here’re the steps on how behavioural targeting technology can be used to minimise fraud risk:
- Behavioural Data Collection: Collects data on a customer's online behaviour, including typing rhythm, mouse movements, and navigation patterns. This data is then used to create a behavioural profile for each customer.
- Behavioural Profiling: The process involves analysing the collected data to identify unique behaviour patterns for each customer. This information is then used to establish a baseline for different kind of typical customer behaviours.
- Real-time Monitoring & Fraud Detection: Continuously monitors customer activity in real-time and compares it to the established baseline. If the system detects any deviations from typical behaviour, it flags the activity as suspicious. The financial institution will receive an immediate alert which enable them to take appropriate action to prevent fraud. A more sophisticated technology can even provide recommendations about the most efficient actions to be taken for different kind of customers or accounts to prevent fraud. For example, recommending the institution to require additional forms of authentication from the customer, such as a one-time code, or may freeze the account until the customer can be contacted.
- Multi-layered fraud detection methodologies that “leave no stone unturned” for all type of frauds. Rule-based systems is still necessary in detecting fraud but it’s not enough. It can be taken as the “first line of defense” especially for the known frauds pattern and more machine learning algorithms or diagnosis can be included in the system such as model-based model, cased-based model, and more. For example, customer A has passed through the rule-based diagnosis, but it will need to also passed through the model diagnosis and the case-based diagnosis to indicate whether the customer is not suspicious at all, having low fraud risk or high fraud risk. This entire process can be just done in seconds.
- An Advanced Scoring Model or Ranking System that enable the banks to prioritise on the fraud prevention tasks. It’s fabulous that you have a well-trained and developed fraud detection and prevention model, but it’s also important that the system interpret the model into scores and/ or ranks. The end users use gain better understanding on which customer or transactions are more likely to be fraudulent so that they are able prioritise on the high score or rank customer transaction and take immediate prevention actions.
- The Use of Network Analysis in bank fraud detection that could analyse the anomaly behaviour correlated across channels and detect organised crime and collusion based on the analysis of the relationship. It’s increasingly important for financial institutions to be able to analyse suspicious transactional frauds as quick as possible since online transactions nowadays can just be done in a few seconds. Network analysis could directly provide a 360-view about the case and the anomaly behaviour in just a few clicks to save investigation time and also improve operational efficiency for the financial institutions to make quick decisions to tackle the frauds.
Preventing Bank Fraud Losses with State-of-the-Art Detection Technologies
Fraudsters are becoming more sophisticated and their methods are evolving, so it is important for the banks and financial institutions to evolve and being scalable to the latest technologies. Using only one of the above strategies is definitely not enough, a well-designed fraud detection system can help banks and financial institutions to reduce their exposure to fraud losses, protect their customers, and maintain their reputation by identifying patterns, detecting anomalies, and making informed decisions in real-time. One of the largest banks in Asia has found their right balance for their fraud detection and prevent system which successfully achieve 50% reduce in false positives and able to detect 30% more types of fraud since 3 years ago and it’s still keeping up until now with minimal fraud risks.
Thus, “crack the code” earlier will definitely enable the banks and financial institutions to be away from any fraud “war" at all time, and it’s possible! Ultimately, having a robust fraud detection system is not only important for banks' bottom line, but also for ensuring the trust and confidence of their customers.
What are you waiting for? Act NOW to enjoy your desired outcome!
Find out more at https://info.kewmann.com/fraud-detection-leave-no-stone-unturned

Macy is a marketing specialist of KewMann and an author of inbound content about technologies related topics. She has five years' experience of business development and marketing in IT industry which covered Big Data, Cloud Computing, Artificial Intelligence, Machine Learning, Behavioural Science and more. She loves to explore new IT knowledge & trends while transforming them into an easy-to-understand blog to share her thoughts with the public.
Subscribe to Email Updates
View more blogs...